BrainChip Discloses Akida, A Neuromorphic SoC
BrainChip, a neuromorphic computing startup has recently disclosed some initial details about their upcoming architecture. BrainChip was founded in 2013, has been listed on the Australian Stock Exchange since 2015, and has raised roughly $30 million through the sale of securities. Last year, they introduced BrainChip Studio, a NN-based video and image analysis software. Earlier this month, they officially announced the Akida Neuromorphic System-on-Chip (NSoC) architecture.
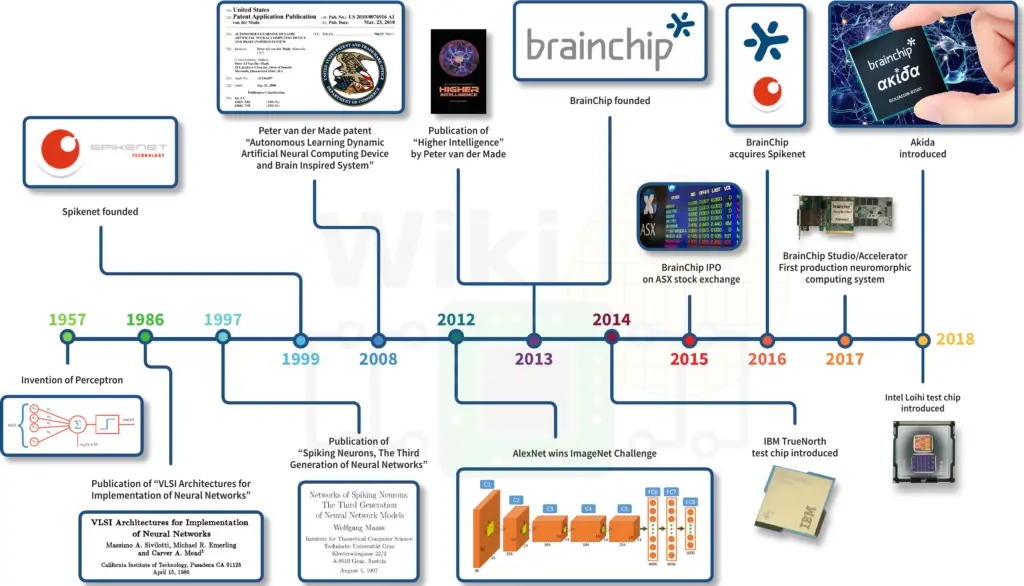
Akida, Greek for ‘spike,’ is a neuromorphic SoC that implements a spiking neural network. In many ways, it’s similar to some of the well-known research projects that were presented over the past several years such as IBM’s TrueNorth, SpiNNaker, and Intel Loihi. With Akida, BrainChip is attempting to seize this early market opportunity with one of the first commercial products.
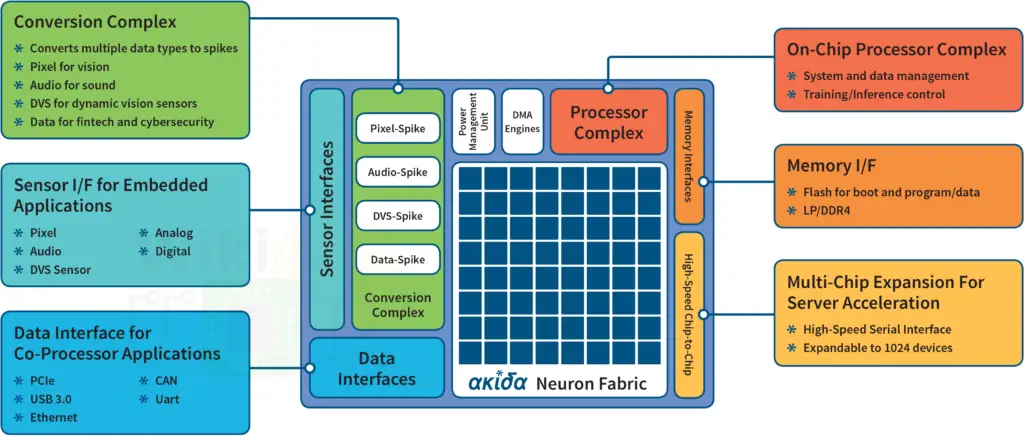
BrainChip is targeting a wide range of markets from the sub-1W edge applications to higher power and performance applications in the data center. Compared to prior research projects, Akida is a complete SoC in the traditional sense. It’s got an on-chip processor complex, memory interfaces, and perhaps more valuable, a wealth of sensor interfaces. Implementing a spiking neural network means it becomes necessary to convert audio, visual, and other types of data into spike form. Akida incorporates a conversion complex designed to convert the acquired data into spikes. Alternatively, for dealing with data that comes from a server (e.g., network traffic, file classification), Akida supports data-to-spikes conversions via data interfaces such as Ethernet, PCIe, and USB. Though the details are a little thin at this time, the conversion complex will play a critical role in making this architecture a success.
SNNs are feed-forward. Neurons learn through selective reinforcement or inhibition of synapses. The Akida NSoC has a neuron fabric comprised of 1.2 million neurons and 10 billion synapses. Sensor data such as images are converted into spikes. From there, clustering results in the neuron fabric picking up repeating patterns which can be used for detection and analysis. For training, both supervised and unsupervised modes are supported. In the supervised mode, initial layers of the network are trained autonomously with the labels being applied to the final fully-connected layer. This makes it possible for the networks to function as classification networks. Unsupervised learning from unlabeled data as well as label classification is possible. BrainChip says training can be done on-chip or off-chip via the Akida Development Environment. For supervised learning, BrainChip sees cybersecurity applications such as malware detection and anomaly detection as some of the initial use cases. At the edge, vision applications can take advantage of the chip sensor interfaces and do accurate object classification.
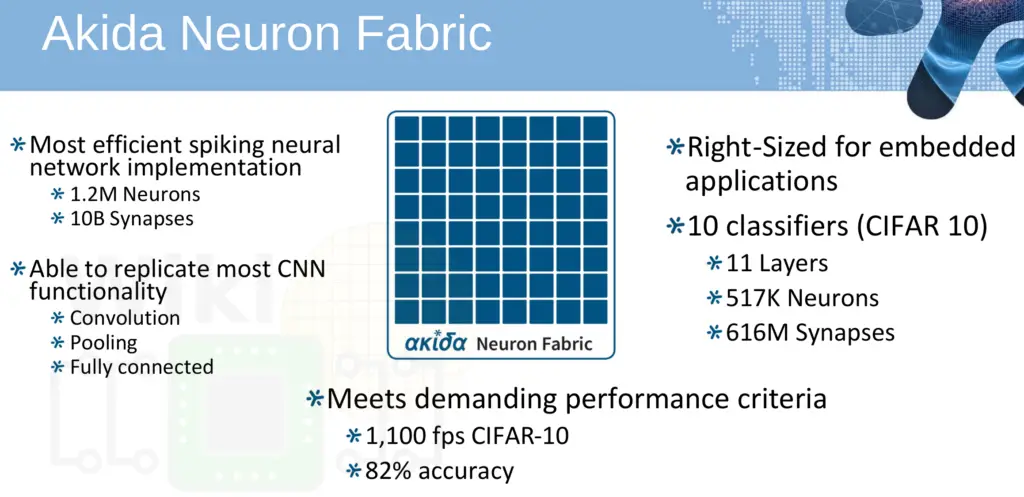
BrainChip is releasing the Akida Development Environment this quarter with FPGA-based accelerator cards available by the end of the year. Akida is process agnostic and the neuron fabric RTL is complete. The company has said they are currently evaluating both 28 nm and 14 nm as possible technologies. Due to this, no pricing is currently being disclosed but the company is aiming for pricing aimed at volume production (presumably around the ten to fifteen dollar range). BrainChip expects samples by Q3 2019 with a multi-chip accelerator card by the end of next year.
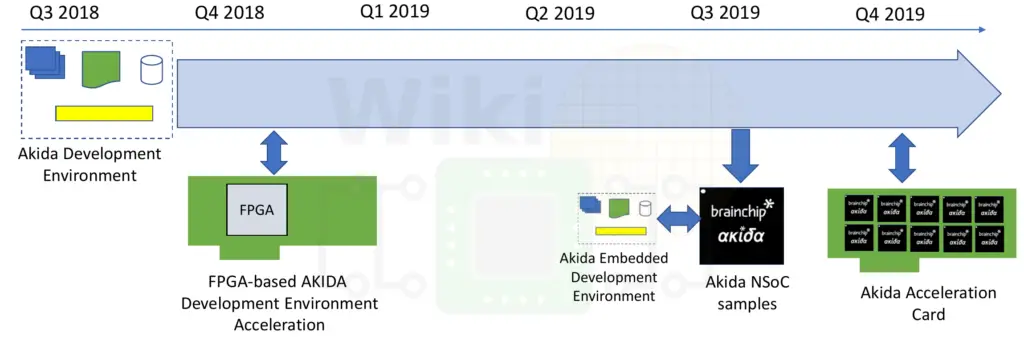
–
Spotted an error? Help us fix it! Simply select the problematic text and press Ctrl+Enter to notify us.
–